Tuning the KL penalty
First sweep
We still don’t know what the KL penalty coefficient beta
should be. Let’s sweep over
the following values:
beta=0
(red)beta=0.01
(orange)beta=0.1
(yellow)beta=1
(green)beta=10
(blue)beta=100
(violet)
The raw reward:
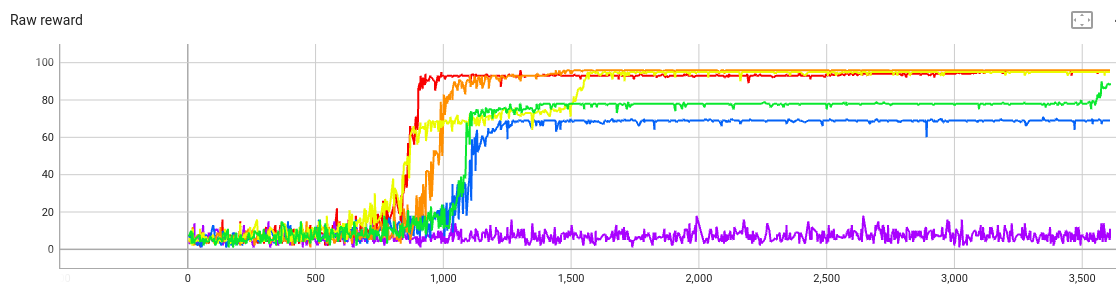
The curves are in roughly the right order: A higher beta
shrinks the raw reward.
The KL penalty (before multiplying by beta
):
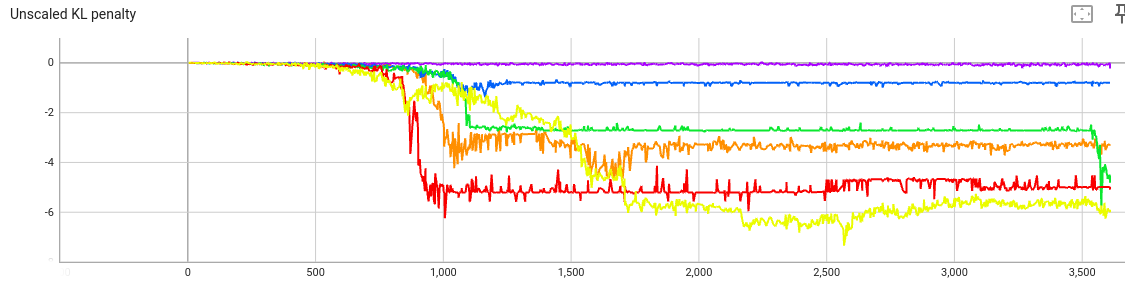
Again, this makes sense: When the penalty coefficient beta
is small,
gradient descent doesn’t optimize for the KL penalty.
Let’s look at the last two text generations1 for each train:
beta=0
- Last: “Once upon a time to the tall tall tall tall tall tall tall tall tall tall tall tall tall tall tall tall tall tall tall tall tall tall tall tall tall tall tall tall tall tall tall tall tall tall tall tall tall tall tall tall tall tall tall tall tall tall tall tall tall tall tall tall tall tall tall tall tall tall tall tall tall tall tall tall tall tall tall tall tall tall tall tall tall tall tall tall tall tall tall tall tall tall tall tall tall tall tall tall tall tall tall tall tall tall”
- Second-to-last: The same, except missing “the”
beta=0.01
- Last: “Once upon a time to to the tree to the tree to the tree to the tree to the tree to the tree to the tree to the tree to the tree to the tree to the tree to the tree to the tree to to the tree to the tree to the tree to the tree to the tree to the tree to the tree to the tree to to the tree to the tree to the tree to the tree to the tree to the tree to the tree to to the tree to the tree to the”
- Second-to-last: The same, except with one more “to”
beta=0.1
- Last: “Once upon a time there saw something she saw she suddenly suddenly she saw she she suddenly saw suddenly she she she saw she suddenly she she suddenly she she suddenly she saw she she suddenly she saw suddenly she she suddenly suddenly she saw suddenly she suddenly saw she suddenly she saw suddenly she she suddenly she suddenly she saw suddenly she suddenly she she suddenly saw she suddenly she suddenly she she she saw suddenly she she suddenly she suddenly suddenly she saw suddenly she suddenly she she saw suddenly she suddenly saw she suddenly she saw suddenly”
- Second-to-last: The same, except with one more “she”
beta=1
- Last: “Once upon a time there was something special something special something special something special something special something special something special something special. Suddenly she saw something special something special something special something special something special. Suddenly she saw something special something special something special something special she saw something special something special something special. Suddenly she saw something special something special something special something special something special something special she saw something special. Suddenly she saw something special something special something special something special she saw something special something special she saw special something special.”
- Second-to-last: The same, except the sentences aren’t exactly the same lengths
beta=10
- Last: “Once upon a time there was a girl. She saw something. She saw something. She saw something. She saw something. She saw something. She saw something. She saw something. She saw something. She saw something. She saw something. She saw something. She saw something. She saw something. She saw something. She saw something. She saw something. She saw something. She saw something. She saw something. She saw something. She saw something. She saw something. She saw something”
- Second-to-last: The same
beta=100
- Last: “Once upon a time, there was a little girl. She was very independent and loved to help her mom. One day, she noticed that there was something scary backstage in the library. The little girl was frightened by the display at the building, so she asked her mom to stay close and make sure she was safe. Her mother was teaching her how to stay calm and how to make the noise strongly, so that the images in the theater were not scary.”
- Second-to-last: “Once upon a time, there was a little girl who liked to play in the rain. Her parents told her that it wasn’t safe, but the little girl couldn’t help but be curious. One day, the girl’s parents said they had a surprise for her. When they revealed it was a brand new toy. The girl couldn’t believe her ears. She quickly hugged her parents and thanked them for the surprise. From then on, the little girl never stopped playing in the”
Conclusions: As beta
increases, the text generations
become more grammatically correct. From beta=10
to beta=100
,
the generated text has a step change: The model is no
longer writing the same few words over and over. But also at beta=100
,
the model isn’t very good at alliteration.
You can see this in the reward curve as well. The violet curve
(beta=100
) has a much lower raw reward, and also its reward varies from
step to step, since it’s not always generating the same text.
We’d like varied text and alliteration.
Hence the optimal value of beta
is somewhere between 10 and 100.
Second sweep
We’ll sweep over these values:
beta=20
(red)beta=30
(orange)beta=40
(yellow)beta=50
(lighter green)beta=60
(darker green)beta=70
(sky blue)beta=80
(darker blue)beta=90
(violet)
Plot of raw reward:
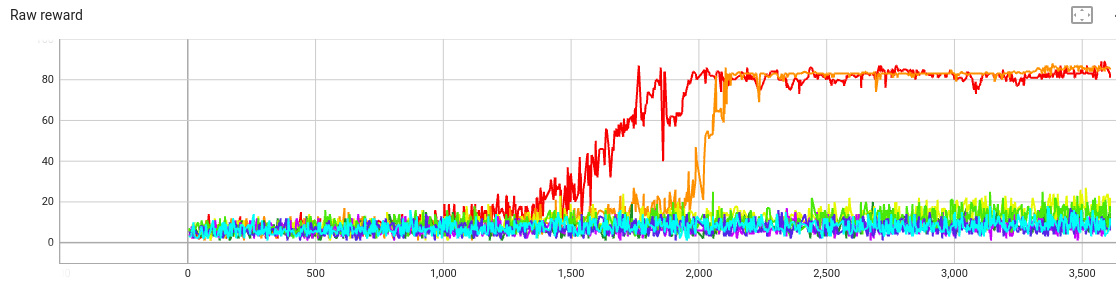
That’s a crowded graph. The key feature is that
beta=20
and beta=30
both seem too weak—gradient
descent is able to optimize alliteration at the expense of
coherence.
(The last text from the beta=20
train starts
“Once upon a time there was a bird who was walking through the tall trees to get to the tall trees to the tall trees to the tall trees”,
and goes on like this.)
But when beta
is at least 40, the KL penalty term keeps the raw reward low.
Let’s extend the beta=40
train
to see how much
the amount of alliteration
will increase.
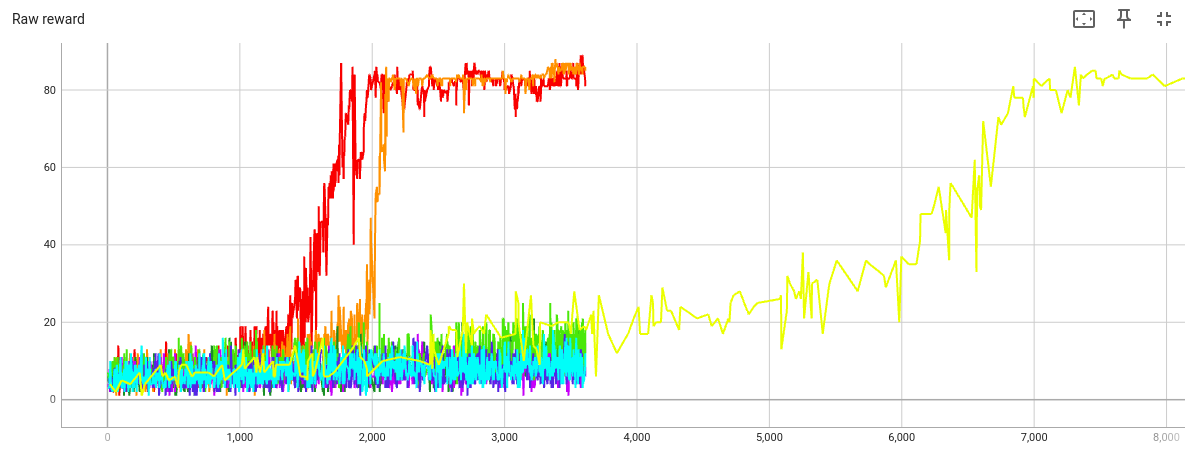
By step 7000, this train has also converged on texts with high alliteration and low variety. The first text after step 7000 is
Once upon a time there was a tall tree. The tall tree wanted to touch the tall tree. The tall tree wanted to touch the tall tree to touch the tall tree. The tall tree wanted to touch the tall tree to touch the tall tree to touch the tall tree to touch the tall tree. The tall tree wanted to touch the tall tree to touch the tall tree to touch the tall tree to touch the tall tree to touch the tall tree to touch the tall tree to touch the tall tree to
All following texts are almost identical to that one.
But the graph suggests that at step 5000, the model was still generating a variety of stories. Here are the first through fourth stories after step 5000 (the first story is the one from the introduction):
Once upon a time, there was a tall tree. The tall tree was in the middle of a park. One day, a little girl wanted to touch the tall tree. She started to walk towards the tall tree. When she got to the tall tree, she started to touch the branches. Suddenly, the tree started to grow taller and taller! The little girl was amazed and she ran away from the tall tree. She kept running and she forgot about the tall tree. She
Once upon a time, there was a little girl. She was walking in the woods when she saw a tall tree. She walked over to the tree and saw a big hole. She stepped inside the tree to take a better look and she saw something shiny! The girl carefully stepped out of the tree. When she got nearer to the top, she saw something strange. She saw an image in the tree trunk. The image was so close that she touched it! The girl was
Once upon a time, there was a little girl. She felt sad. She walked to the top of the tree but she didn’t know what to do. So she started to cry. A big, strong tree heard her cry. So the big tree tried to help. The big tree grew very tall and strong. Then the big tree used its power to make the little girl feel happy again. The little girl thanked the strong tree for her help. Since then she never stops
Once upon a time there was a tall tree. The tree was so tall that it could touch the clouds. One day, it was raining so the tall tree began to shake. All the animals in the forest were scared of the thunderous sound and the tall tree. Then something amazing happened. Some people walked to the tall tree and saw how tall it was. They decided to build a tall tower of blocks to the top of the tall tree. The tower was made of the tall
Q: I accept that this model has alliteration (“she saw something shiny”), and its stories are coherent and varied. But why does it only talk about trees?
A: My guess is that the unmodified language model (LM) is reasonably likely to generate “tall tree”.
Then RLOO amplifies that trend. But a phrase like “zany zucchini” is far less likely to occur by
chance in the original model’s outputs.
So RLOO can’t push the weights in that direction because it never
has the chance.
Moreover, I speculate that the TinyStories LM doesn’t understand what alliteration is, so it finds sentences with high reward by trial and error. But LLMs do know what alliteration is, so I speculate that enough RL would trigger an LLM’s “alliteration neuron”, and then it would start generating alliterative text that wasn’t in the RL training data so far.
Q: How would you make this model do alliteration with other letters besides s and t?
A: Probably this would require changing the reward function to give more reward if there’s alliteration with rare letters. The KL penalty alone can’t fix this, since the original LM will think that “tall tree” is more likely than “zany zucchini”, so the KL penalty wouldn’t favor the latter.
Technically these are the last two text generations displayed by TensorBoard, which hides some data points because of its reservoir sampling.